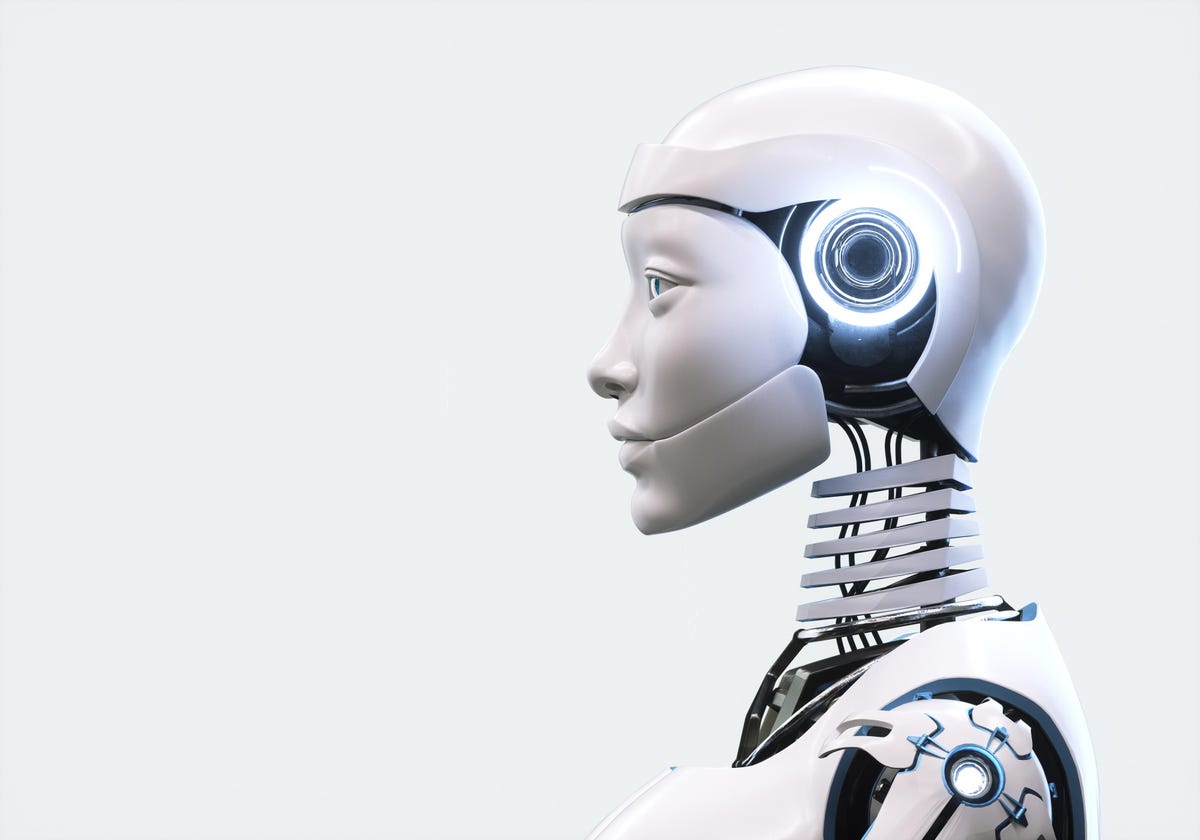
Federated learning is an approach to machine-learning that trains an algorithm across multiple decentralized edge servers and devices, each storing local samples of data. Federated Learning does not permit data exchange between edge servers and devices. While the applications are based on simple logic, stateful computation and secure aggregation, they do not work in federated learning. In certain cases, data can be obtained from more than one source. Federated learning is an excellent choice for machine-learning apps.
ML applications work on simple logic
While most ML applications use simple logic, complex real-world problems often require highly specialized algorithms. These problems include "is there cancer?" ", "What did I say?" and other tasks that require perfect guesses. Fortunately, there are several real-world applications of machine learning. This article gives an overview of machine learning and how it can be applied to these areas. It also contains a discussion on how it can help reduce labour costs.
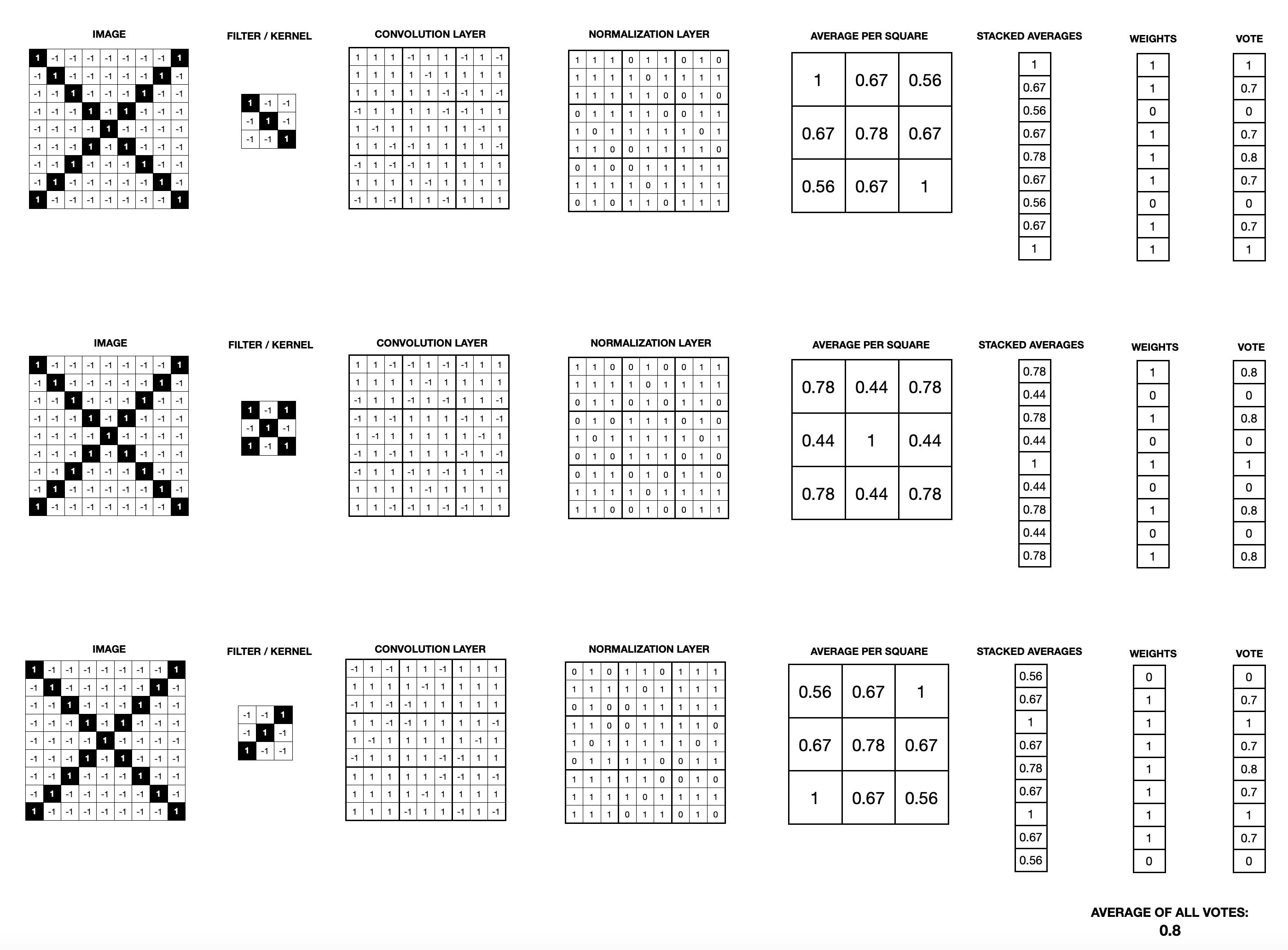
ML applications rely on stateful computations
The main question in ML is "how can federated ML applications function?" This article will discuss the key principles and practical issues of federated learning. In federated learning, stateful computations are used in multiple data centers. Each data centre contains thousands of servers that each run a different ML algorithm. These two types of stateful computations, stateful and unreliable, are both highly unpredictable. In stateless computations, clients have a fresh sample of data to process in each round, while highly unreliable computing assumes that 5% or more of clients are down. Clients have the ability to arbitrarily divide data. The data can be partitioned vertically or horizontally. Topology refers to a hub-and-spoke network with a coordinator service provider at its center and spokes.
A server initializes the global model for a federated learning system. The global model is then sent out to clients. Each device then updates its local model. Once client devices have updated their local versions, the server then aggregates and applies the data to the global model. This process is repeated numerous times. The final result is the simple sum of all the local models.
ML applications work on secure aggregation
FL is still early in development, but it is already proving its worth as a data-based alternative to machine learning. This type of learning framework does away with the need to collect and upload user-generated data, which can raise privacy concerns. This kind of learning can also be used to learn without data or labels. If there are security precautions, it can be integrated into everyday products. FL is still an area of interest.
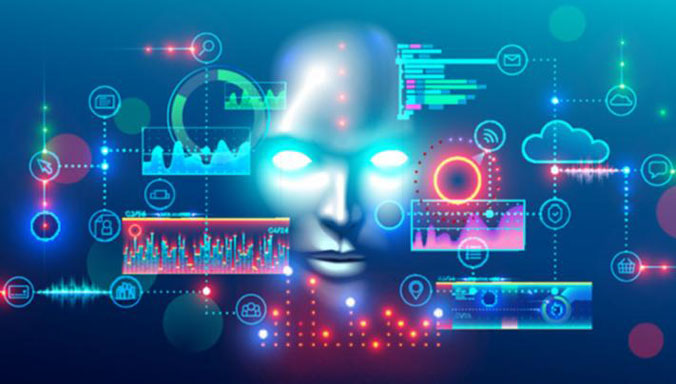
FL is an effective and safe way to gather local machine learning results. It can be used to improve the search suggestions in Gboard. It utilizes a client/server architecture for distributing ML tasks among multiple devices. The algorithms are executed by the clients and sent back to the server. Researchers also addressed battery-usage and network communication issues with FL. Finally, they addressed the issue of ML model updates, which often sabotaged the ML training process.
FAQ
Which industries use AI most frequently?
The automotive industry was one of the first to embrace AI. BMW AG uses AI as a diagnostic tool for car problems; Ford Motor Company uses AI when developing self-driving cars; General Motors uses AI with its autonomous vehicle fleet.
Other AI industries include banking, insurance, healthcare, retail, manufacturing, telecommunications, transportation, and utilities.
Is Alexa an Artificial Intelligence?
Yes. But not quite yet.
Amazon developed Alexa, which is a cloud-based voice and messaging service. It allows users interact with devices by speaking.
The Echo smart speaker was the first to release Alexa's technology. Since then, many companies have created their own versions using similar technologies.
These include Google Home, Apple Siri and Microsoft Cortana.
Are there potential dangers associated with AI technology?
It is. There will always exist. AI poses a significant threat for society as a whole, according to experts. Others argue that AI can be beneficial, but it is also necessary to improve quality of life.
AI's greatest threat is its potential for misuse. It could have dangerous consequences if AI becomes too powerful. This includes robot overlords and autonomous weapons.
Another risk is that AI could replace jobs. Many people are concerned that robots will replace human workers. Others think artificial intelligence could let workers concentrate on other aspects.
For instance, some economists predict that automation could increase productivity and reduce unemployment.
What is the newest AI invention?
Deep Learning is the most recent AI invention. Deep learning is an artificial intelligent technique that uses neural networking (a type if machine learning) to perform tasks like speech recognition, image recognition and translation as well as natural language processing. Google invented it in 2012.
Google's most recent use of deep learning was to create a program that could write its own code. This was done using a neural network called "Google Brain," which was trained on a massive amount of data from YouTube videos.
This allowed the system's ability to write programs by itself.
IBM announced in 2015 the creation of a computer program which could create music. Music creation is also performed using neural networks. These are called "neural network for music" (NN-FM).
Statistics
- According to the company's website, more than 800 financial firms use AlphaSense, including some Fortune 500 corporations. (builtin.com)
- Additionally, keeping in mind the current crisis, the AI is designed in a manner where it reduces the carbon footprint by 20-40%. (analyticsinsight.net)
- A 2021 Pew Research survey revealed that 37 percent of respondents who are more concerned than excited about AI had concerns including job loss, privacy, and AI's potential to “surpass human skills.” (builtin.com)
- In 2019, AI adoption among large companies increased by 47% compared to 2018, according to the latest Artificial IntelligenceIndex report. (marsner.com)
- More than 70 percent of users claim they book trips on their phones, review travel tips, and research local landmarks and restaurants. (builtin.com)
External Links
How To
How to configure Siri to Talk While Charging
Siri can do many tasks, but Siri cannot communicate with you. Your iPhone does not have a microphone. Bluetooth is a better alternative to Siri.
Here's how to make Siri speak when charging.
-
Select "Speak When locked" under "When using Assistive Touch."
-
To activate Siri, press the home button twice.
-
Siri can speak.
-
Say, "Hey Siri."
-
Simply say "OK."
-
Speak: "Tell me something fascinating!"
-
Say "I'm bored," "Play some music," "Call my friend," "Remind me about, ""Take a picture," "Set a timer," "Check out," and so on.
-
Speak "Done."
-
Thank her by saying "Thank you"
-
If you are using an iPhone X/XS, remove the battery cover.
-
Replace the battery.
-
Reassemble the iPhone.
-
Connect the iPhone with iTunes
-
Sync the iPhone
-
Allow "Use toggle" to turn the switch on.